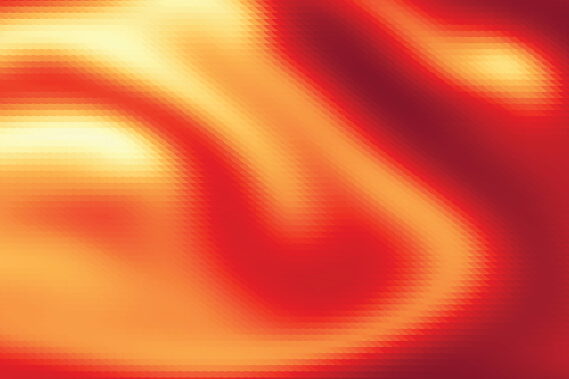
Artificial intelligence allows us to see the plasma edge of fusion in new ways
Researchers must understand how plasmas move in order to make fusion energy an economically viable resource. This is a mixture of electrons and ions swirling in reactor vessels. To make fusion devices produce significant gains in net power, the plasma particles must be contained in magnetic fields in toroidal chambers called tokamaks. This is difficult because the plasma’s hot edge (which is over 1 million degrees Celsius) lies only centimeters from the vessel’s cooler solid walls.
Abhilash Mathews is a PhD candidate at the Department of Nuclear Science and Engineering at MIT’s Plasma Science and Fussion Center (PSFC). He believes that this plasma edge provides a wealth of unanswered questions. It is a turbulent boundary that is crucial to understanding plasma confinement and fueling. Also, heat fluxes can strike surfaces of materials, which can have a detrimental effect on fusion reactor designs.
Scientists focus on understanding edge conditions by modeling turbulent flow at the boundary. This is done using numerical simulations to help predict plasma behavior. These “first principles” simulations are the most difficult and time-consuming in fusion research. Researchers could accelerate progress by developing “reduced” computer model that runs much faster and has quantifiable levels of accuracy.
Tokamak physicists used a lower “two-fluid” theory to simulate boundary plasmas in experiments for decades. However, there has been uncertainty about their accuracy. Mathews has published two recent papers that directly test the accuracy of this reduced plasma-turbulence model. He combines physics and machine learning.
Mathews explains that a successful theory should be able to predict what you will observe. “For example, the temperature and density, the electric potent, and the flows. These relationships are what define a turbulence theory. Our work examines the dynamic relationship between the turbulent electric field (the electron pressure) and the turbulent electrical field (the electron force).
Mathews uses a new deep-learning technique to use artificial neural networks to create representations of equations that govern the reduced fluid theory in the first article, published Physical Review. Mathews uses this framework to demonstrate a method of computing the turbulent electric field in plasma from electron pressure fluctuations. This is consistent with the reduced fluid theorem. While models are commonly used to link the electric field and pressure, this model is robust to noisy pressure measurements.
Mathews investigates the connection further in the second paper published in Physics of Plasmas. He compares it with higher-fidelity simulations of turbulence. It was previously difficult, if not impossible, to accurately evaluate turbulence between models. Mathews found that plasmas relevant for existing fusion devices are compatible with the reduced fluid model’s predicted turbulent field. This is consistent with high-fidelity calculations. This is why the reduced turbulence theory does work. Mathews says that it is important to verify the theory by examining every connection between variables.
Principal Research Scientist Jerry Hughes is Mathews’ adviser. He notes that plasma turbulentity is more difficult than the turbulence in air or water. “This research shows that physics-informed machine learning techniques can provide a complete picture of rapidly fluctuating edge plasma starting from a small set of observations. This is exciting to me because we don’t always have the ability to observe all quantities.
These physics-informed deep learning methods open up new avenues for testing theories and expanding the scope of what can be seen from new experiments. These applications, according to David Hatch, a researcher at the Institute for Fusion Studies at The University of Texas at Austin believe that they are the beginning of a promising new technology.
He says that Abhi’s work “is a major accomplishment with the potential to have broad applications.” “Physics-informed machine learning could, for example, infer additional plasma quantities from a nearby domain if there are only limited diagnostic measurements of a particular plasma quantity. This would augment the information provided by a diagnostic. This technique opens up new avenues for model validation.
Mathews anticipates exciting research.
He says, “Translating these methods into fusion experiments to real edge plasmas (one goal) is something we have in view, and work is currently underway.” “But this is only the beginning.”