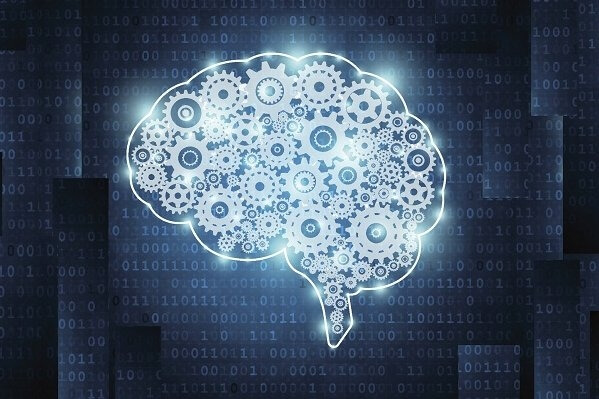
Machine learning in healthcare: The downside
Marzyeh Hassemi was working towards her doctorate in computer science at MIT. She wrote several papers about how machine-learning techniques could be applied in clinical data to predict patient outcomes. “It was only after I finished my PhD that one of my committee members asked me: “Did your model work across different groups of patients?”
Ghassemi was shocked to see the results of this question. She had evaluated models across all patients and had previously examined their performance in aggregate. She was shocked to discover that models can often work differently for Black women. She says, “I didn’t know that model disparities could be linked to health disparities.” “And because I am a visible minority woman-identifying computer scientist at MIT I’m reasonably certain that others didn’t know this.”
In a paper published Jan. 14, in the journal patterns,Ghassemi, who received her doctorate in 2017, and is now assistant professor in the Department of Electrical Engineering and Computer Science and MIT Institute for Medical Engineering and Science, (IMES), offer a warning about the potential for AI in medicine. Ghassemi states that if used properly, this technology can improve health care performance and possibly reduce inequities. Technology could make care worse if we aren’t careful.”
Data is the key, as AI tools train by analyzing and processing large amounts of data. However, the data they receive are generated by humans who are human and can make mistakes. They may also interact with patients differently depending on their gender, age and race.
There is also a lot of uncertainty surrounding medical conditions. Ghassemi states that doctors who have been trained at the same medical school 10 years ago can disagree on a patient’s diagnosis. This is different than the applications in which existing machine-learning algorithms excel, such as object-recognition tasks. Because almost everyone on the planet will agree that a dog, in fact, is a dog, this is not an exception.
Machine-learning algorithms also have performed well in learning how to play games such as chess or Go. This is because both the rules and “win conditions” of the game are clearly defined. However, physicians don’t always agree on the rules of treating patients and the win condition of “healthy” isn’t widely accepted. Ghassemi says that doctors know what it is to be sick and have the best data when people are most sick. We don’t have much data when people are healthy, because they are less likely to visit doctors.
Even machines can cause errors and inaccuracies in treatment. For example, pulse oximeters that were calibrated primarily on people with light skin do not accurately measure blood oxygen levels in those with darker skin. These deficiencies are especially acute when oxygen levels drop — when precise readings are needed most. Ghassemi & Nsoesie also note that women are at greater risk during “metal on metal” hip replacements. This is due in part to anatomical differences that aren’t considered in implant design. These facts could be hidden in the data feed to computer models, whose output will be compromised.
According to Ghassemi, machine-learning algorithms offer “the sheen” of objectivity because they are generated from computers. This can be dangerous and deceptive. It’s much harder to spot the errors in the data that is being sent to computers en masse than to disregard the advice of one doctor, who may be inept or even racist. She insists that machine learning is not the problem. “It’s people. Sometimes, human caregivers produce bad data because they’re not perfect.
She believes machine learning can bring benefits to health care, with more effective and fair recommendations and practices. The key to realizing machine learning’s promise in health care is improving the quality of data. This is not an easy task. Ghassemi explains, “Imagine if data could be taken from doctors with the highest performance and shared with doctors who have less experience and training.” We must collect and audit this data.”
She notes that it is difficult to get data collected in a way that is rewarding or incentivised. It’s difficult to get grants for this or to ask students to dedicate time to it. Data providers may argue, “Why should I give out my data for free when I could sell it to a business for millions?” But researchers should have the ability to access data without needing to answer questions such as: “What paper will you get my name on in return for accessing data that is at my institution?”
Ghassemi states that “the only way to improve health care is to obtain better data.” He also says that incentivizing data release is the best way to get better information.
It’s more than just about collecting data. It’s not just about collecting data. Ghassemi suggests assembling diverse research groups — statisticians, clinicians, medical ethicists and computer scientists — to gather patient data first and then “focus upon developing fair and equitable improvements for health care that can not only be used in one advanced medical setting but in a variety of medical settings.”
She says that the Patternspaper’s purpose is to encourage technologists to bring their machine learning expertise to the medical community. They just need to be aware of the limitations in treatment and other complexities before they approve a specific computer model.