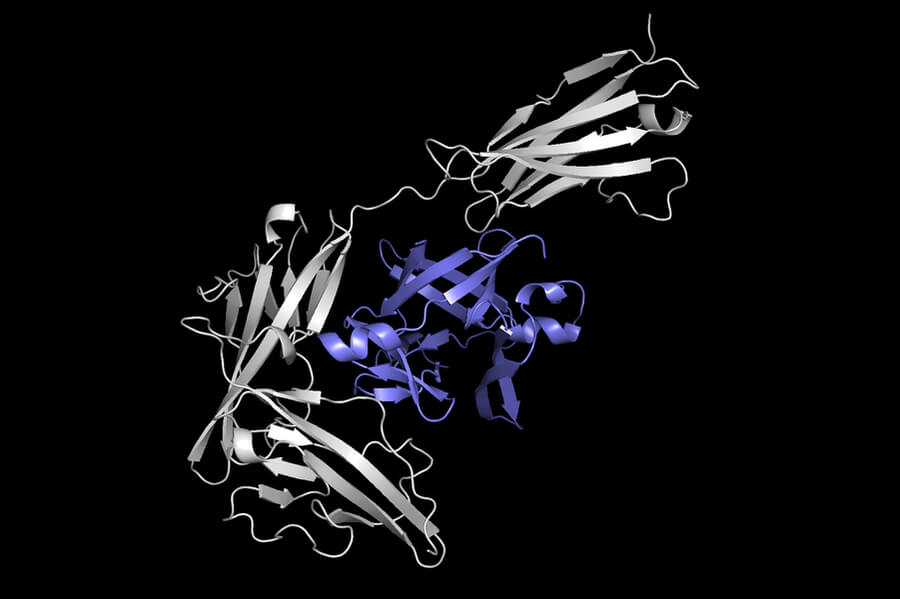
Artificial intelligence system quickly predicts which two proteins will be attached
Small proteins called antibodies, which are small proteins made by the immune system can attach to certain parts of viruses to neutralize them. Scientists continue to fight SARS-CoV-2 the virus that causes Covid-19. One possible weapon is a synthetic antibody that binds to the spike proteins of the virus to stop it from entering human cells.
Researchers must be able to understand how synthetic antibodies attach in order to develop them. It is difficult to find the right protein complex from a multitude of candidates because proteins have complicated 3D structures that can be glued together with many folds.
MIT researchers developed a machine learning model that predicts the structure of complexes that form when two proteins bind together. This was done to speed up the process. Their method is between 80-500 times faster than current software and predicts proteins structures that are closer in relation to experimentally observed structures.
This technique could be used to help scientists understand biological processes, such as DNA replication and repair. It could also accelerate the development of new medicines.
Deep Learning captures interactions between proteins in a way that is easy for biologists and chemists to experiment with experimentally. These interactions can be very complex and have not been explained in a clear way. “This deep-learning model is able to learn these types of interactions using data,” says Octavian Eugen Ganea, a postdoc at the MIT Computer Science and Artificial Intelligence Laboratory. He was also co-lead author.
Xinyuan, a graduate student from ETH Zurich, is Ganea’s colead author. Regina Barzilay, a School of Engineering Distinguished Professor for AI and Health at CSAIL, and Tommi Jaykola, the Thomas Siebel Prof of Electrical Engineering at CSAIL and a member of Institute for Data, Systems, and Society, are co-authors of MIT. The research will be presented during the International Conference on Learning Representations.
Protein attachment
Equidock is the model developed by researchers. It focuses on rigid body Docking, which occurs when two proteins attach to each other in 3D space. However, their shapes don’t bend or squeeze.
The model converts the 3D structures of 2 proteins into 3D graphs which can be processed by a neural network. Each amino acid is represented as a node within the graph. Proteins are made from chains of amino compounds.
Researchers incorporated geometric knowledge into their model to understand how objects change when they are rotated in 3D space. Mathematical knowledge is also included in the model to ensure that proteins attach the same way no matter where they are located in 3D space. This is how proteins attach to the human body.
This information is used by the machine-learning system to identify the atoms of two proteins most likely to interact with each other and create chemical reactions. These points are known as binding pockets. These points are used to combine the proteins into a complex.
“If we can identify which parts of the proteins are likely to have these binding pockets points, we will be able to put the two proteins together. Ganea says that if we find the two sets of points, we can then translate and rotate the proteins to match the other set.
The lack of data for training was one of the greatest challenges in building this model. Equidock was created because there is so little experimental 3D data available for proteins. Ganea states that it was crucial to include geometric knowledge. The model could pick up false correlations without these geometric constraints.
Seconds vs. Hours
The researchers compared the model to four different software programs after it was trained. Equidock can predict the final protein complex in as little as one to five seconds. The baselines took longer than that, taking between 10 and an hour.
Equidock often performed well in quality measures that measure how close the predicted protein complex matches actual protein complexes. However, it was sometimes less than the baselines.
“We still trail behind one of our baselines. It is possible to improve our method, but it could still prove useful. It could be used for large-scale virtual screenings, where thousands of proteins interact to form complexes. He says that our method could be used to quickly generate a set of candidates, which could then be refined with more precise, but slower traditional methods.”
The Equidock team plans to include specific atomic interactions in Equidock to make better predictions. Hydrophobic interactions are the interaction of water molecules with atoms.
Ganea suggests that the same technique could be used to develop small, drug-like molecules. This type of molecules bind to protein surfaces in particular ways. It is possible to quickly determine how this attachment happens, which could reduce the time it takes for drug development.
They plan to improve Equidock in the future so that it can predict flexible protein docking. Ganea and his coworkers are currently working on synthetic data to help improve the model.